GENERAL
Black and White Differences in Cognitive Function Test Scores: What Explains the Difference?
Published
4 years agoon
By
Joe Pee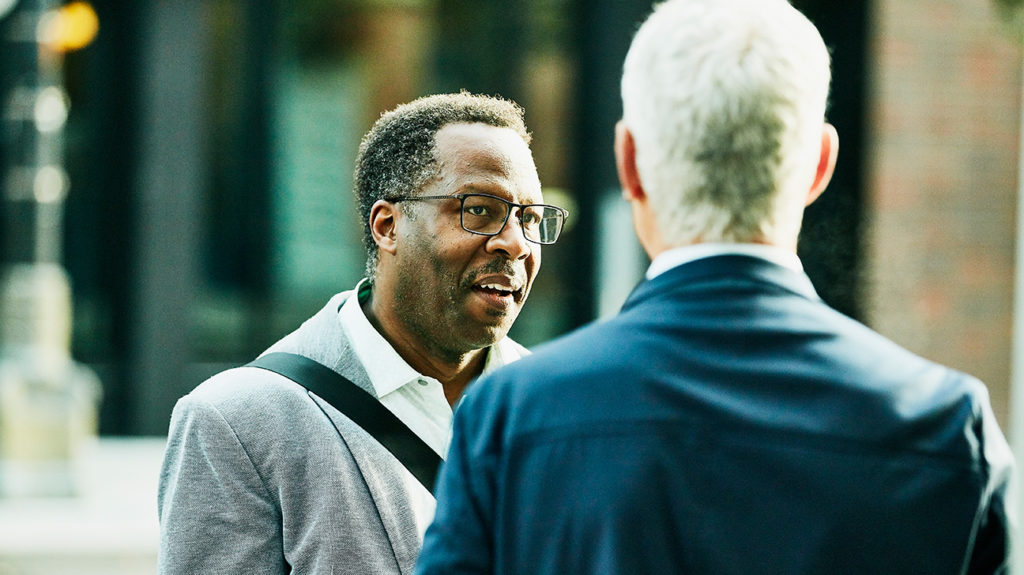
Several studies have reported that older black and Latino adults have lower cognitive function test scores than older white adults, but few have comprehensively examined reasons for score differences. This study evaluates whether differences in health and socioeconomic indicators, including literacy level, can explain differences in cognitive function test scores between older black and white adults.
Participants from the Health, Aging and Body Composition (Health ABC) study, a study of 3,075 adults aged 70 to 79 (mean age 74, 52% female, 42% black) were evaluated. Participant characteristics and performance on two cognitive function tests (the modified Mini-Mental State Examination (3MS) and Digit Symbol Substitution test (DSS)) were assessed in 1997–98. Significant correlates on bivariate tests (α = 0.10) were sequentially added in blocks (demographic, psychosocial, health-related, and socioeconomic) to generalized linear models, with 3MS or DSS score as the dependent variable.
Mean raw 3MS score was 7 points lower in black than white participants (86.0 vs 93.0; P<.001). After adjustment for demographic, psychosocial, health-related, and socioeconomic characteristics, the 7-point difference was reduced to 1 point. Thus, covariate adjustment explained 86% of the difference in 3MS scores between blacks and whites (P<.001). Socioeconomic indicators, particularly literacy, education, and income, explained most of the differences in cognitive scores.
Similar results were found using the DSS. Findings suggest that participant characteristics not only independently affect cognitive test scores, but they also explain most of the observed difference in cognitive performance between older blacks and whites. Given that socioeconomic status indicators, literacy level in particular, may reflect early-life learning experiences, poor cognitive test performance may not reliably indicate cognitive decline in economically disadvantaged populations.
Several studies report differences in cognitive test scores across ethnic groups, with older black and Latino adults having lower scores than older white adults.1–4 A recent study conducted in participants from the Cardiovascular Health Study suggests that rates of dementia also vary between blacks and whites, with the prevalence being higher in black men (22.2%) and women (23.4%) than in white men (13.5%) and women (14.5%).5,6 Some suggest that the validity of tests used to measure cognitive performance and screen for dementia may vary by population subgroup and have low specificity in nonwhite elders.7
Others report that discrepancies on cognitive test scores may be attributed to early-life or life-course disadvantages in terms of education quality8,9 or meaningful differences in health status and the presence and severity of diseases and conditions known to influence cognitive performance.10,11 Few studies have had adequate data on socioeconomic status (SES) and health-related factors to begin to disentangle social versus health effects.
This study’s primary hypothesis was that differences in cognitive test scores between black and white elders stem from variations in psychosocial, health-related, and socioeconomic factors. The aim was to explore how these characteristics might mediate the observed differences in cognitive function test scores between older black and white adults using data from the Health, Aging and Body Composition (Health ABC) study. This cohort is ideally suited to address differences between older black and white adults’ cognitive performance because it consists of more than 3,000 well-functioning community-resident men and women aged 70 to 79 of whom 42% are black. In addition, key explanatory characteristics were carefully measured at baseline or in the early follow-up years. The goal was to identify the magnitude of differences in cognitive function test scores between black and white elders and to determine the degree to which health-related, psychosocial, and socioeconomic factors reduce or eliminate these differences.
METHODS
Study Population
The details of the Health ABC study have been outlined previously.12 In brief, Medicare beneficiary lists were initially used to identify 3,075 older adults aged 70 to 79 at two field centers in Pittsburgh, Pennsylvania, and Memphis, Tennessee. Recruitment from the entire black community was needed at both sites to meet recruitment goals. All participants signed informed consent at each clinical center, and the institutional review board at each institution approved the study. Participants were well functioning at baseline in 1997–98, with exclusion criteria including reported difficulty with walking one-quarter of a mile, climbing 10 steps without resting or performing basic activities of daily living, or requiring a walking aid to ambulate.
Cognitive Function Measurement
At study enrollment, participants completed an interview and a clinical examination that included two examiner-administered cognitive function tests. The Modified Mini-Mental State Examination (3MS) assessed global cognitive function on a scale from 0 to 100, with a higher score indicating better cognition.13 The Digit Symbol Substitution test (DSS) measured attention, psychomotor speed, and executive function. The DSS score was calculated as the total number of test items correctly coded in 90 seconds. Thus, a higher score indicated better cognitive function.14 The analytic sample included the 3,062 participants with complete 3MS information or the 3,032 participants with complete DSS information. In addition to using 3MS as a continuous outcome, low 3MS score was defined as 80 points or below. This cutoff is standard and clinically relevant.15
Demographic and Socioeconomic Characteristics
Baseline characteristics were grouped into four main categories: demographic, psychosocial, health-related, and socioeconomic. Demographic characteristics (age, sex, race) were ascertained through self-report. SES included years of education, family income (<$10,000, $10,000–25,000, >$25,000–50,000 and >$50,000), and perceived income adequacy (whether income met financial needs, had enough money for food, and had money left at the end of each month). At Year 3, literacy level was measured using the Rapid Estimate of Adult Literacy in Medicine (REALM), in which participants were asked to read aloud from a word list of medical terms with varying degrees of difficulty such as “germs” and “obesity.” Scores are based on the number of words read and pronounced correctly, for a total of 0 to 66 points. Higher scores indicate higher literacy levels.16 Because the REALM was not administered until Year 3, only participants who had an in-clinic visit in Year 3 had a literacy assessment, leaving 18% with no information on literacy. To retain the largest sample possible, literacy score was imputed using results from a regression equation that included years of education, sex, study site, and age in the 82% of participants with complete data. The regression equation had adequate fit (coefficient of determination (r2) =0.34). Participants missing literacy score had lower education level, older age, and included more women than participants with literacy scores.
Health-Related and Psychosocial Characteristics
Health-related characteristics included current cigarette smoking, alcohol use (seven drinks per week or more), and physical inactivity (measured as expending less than 200 kcal per week including exercise, walking, and recreational activity). An inventory of all medications (prescription and over the counter) used in the previous 2 weeks was taken using Iowa Drug Information System codes to identify each medication.17 Using the medication inventory, all prescribed and over-the-counter nonsteroidal anti-inflammatory drugs were identified. In addition, standardized algorithms were used to assess prevalent myocardial infarction (by self-report and medication use), cerebrovascular disease (by self-report), hypertension (by sphygmomanometer), and diabetes mellitus (by self-report or medication use) as predictor variables. Body mass index was also calculated as weight in kilograms divided by height in meters squared. A cutoff of 30 was used to indicate obesity.
Psychosocial characteristics were assessed using standardized questions.18 The frequency of social contact was dichotomized according to whether they had social contact less than once per week or more often. Personal mastery beliefs were determined from level of agreement/disagreement with the following statements “I often feel helpless,” and “I can do anything I set my mind to.” Anyone who expressed anything other than strong disagreement with the first statement and strong agreement with the second were considered to have low mastery.19 Similarly, participants who reported that they agreed or strongly agreed with “Do you feel you need more emotional support?” were classified as needing more emotional support. Participants were also asked to rate their health, and this was classified into fair or poor versus good, very good, or excellent. Religious faith was evaluated using the question “How important is your religious faith to you?” Persons who responded that religious faith was “not important” were considered to have low religiosity. Depressive symptoms were measured using the Center for Epidemiologic Study Depression Scale (CES-D), with a higher score indicating presence of more depressive symptoms. CES-D score was used as a continuous variable.20
Statistical Analyses
Chi-square analyses or t tests were conducted to assess whether differences existed in demographic, psychosocial, health-related, and socioeconomic characteristics between black and white participants. Mean, unadjusted scores on cognitive tests (3MS, DSS) were calculated overall and separately in blacks and whites. Any characteristic associated (P<.10) with 3MS or DSS was included in subsequent models in blocks according to the above-described demographic, psychosocial, health-related, and socioeconomic categories. The first set of models examined the association between black race and test score in response to the addition of the covariate blocks, and standardized beta coefficients were calculated. These models used 3MS and DSS score as the dependent variables in multivariate generalized linear models (GLMs). Potential explanatory variables included demographic, psychosocial, health-related and sociodemographic blocks added hierarchically to the GLM models described above.
The second set of GLM models was calculated to determine the source of variance in cognitive test scores. A least squares adjusted mean 3MS score was calculated for blacks and whites separately using each GLM model, and potential explanatory variables were added according to blocks in the following manner: demographic, demographic and psychosocial, demographic and health-related, demographic and socioeconomic, and all four blocks. A similar strategy was used to examine the outcome of low 3MS score (<80 points) using logistic regression models. All analyses were performed using SAS version 8.0 (SAS Institute, Inc., Cary, NC).
RESULTS
Participants were, on average, 74 years old. Black and white participants were similar in age, but black participants were more likely to be female and from the Pittsburgh site (Table 1). Black older adults reported a greater need for emotional support and more often rated their health as fair or poor and lived alone. Black older adults also had higher rates of medical comorbidities, with the exception of myocardial infarction history. Black participants also had lower socioeconomic status (education, literacy scores, income, financial adequacy) than their white counterparts.
Table 1
Baseline Characteristics of the 3,062 Black and White Health, Aging and Body Composition Participants
Characteristic* | Blacks (n = 1,271) | Whites (n = 1,791) | P-value† |
---|---|---|---|
Demographic | |||
Mean age ± SD | 73 ± 3 | 74 ± 3 | .83 |
Female, % | 57 | 48 | <.001 |
Pittsburgh site, % | 52 | 48 | .02 |
Psychosocial | |||
Feels needs a lot of emotional support, % | 18 | 13 | <.001 |
Fair/poor self-rated health, % | 27 | 8 | <.001 |
Low personal mastery beliefs, % | 21 | 23 | .12 |
Lives alone, % | 36 | 26 | <.001 |
Low social contact, % | 80 | 81 | .34 |
Religious faith important, % | 95 | 84 | <.001 |
Center for Epidemiologic Study Depression Scale score, mean ± SD (range 0–43) | 4.8 ± 5 | 4.7 ± 6 | .04 |
Health-related, % | |||
Current smoking | 18 | 8 | <.001 |
≥Seven alcoholic drinks/wk | 5 | 9 | <.001 |
Inactive (<200 kcal/wk) | 46 | 28 | <.001 |
Myocardial infarction | 13 | 13 | .87 |
Stroke/transient ischemic attack | 8 | 6 | .05 |
Hypertension | 74 | 57 | <.001 |
History of diabetes mellitus | 21 | 11 | <.001 |
Obese (body mass index >30) | 36 | 18 | <.001 |
Nonsteroidal antiinflammatory drugs | 22 | 22 | .98 |
Socioeconomic | |||
Education, years, mean ± SD | 12 ± 5 | 14 ± 3 | <.001 |
Literacy, REALM score Year 3, mean ± SD‡ | 55 ± 15 | 64 ± 5 | <.001 |
Literacy, REALM imputed score, mean ± SD‡ | 55 ± 14 | 64 ± 5 | <.001 |
Family income, $, % | |||
<10,000 | 26 | 4 | <.001 |
10,000–25,000 | 49 | 32 | |
>25,000–50,000 | 20 | 40 | |
>50,000 | 5 | 24 | |
Financial inadequacy, % | 20 | 7 | <.001 |
SD = standard deviation.
Both black and white participants had a wide distribution of cognitive scores. On average, black participants had lower unadjusted scores on cognitive function tests than white participants. For example, mean ± standard deviation 3MS scores were 7 points lower in black (86.0 ± 9.7) than white participants (93.0 ± 5.8) (P<.001). Similarly, on average, whites (41.5 ± 11.6) scored 14 points higher on the DSS than blacks (27.9 ± 14.1) (P<.001).
To test the effect that each block had on the magnitude of the black/white difference in cognitive function test scores, standardized beta coefficients associated with ethnicity are presented in successive regression models (Tables 2 and and3).3). The standardized beta remained relatively unchanged until the socioeconomic factors were added as a block and were thereby associated with the black/white difference in 3MS and DSS scores. The standardized beta for black ethnicity was −0.43 with age, sex, and study site in the model and remained virtually unchanged when psychosocial and health-related blocks were added (−0.40 and −0.38, respectively), but when socioeconomic factors were added, the standardized beta decreased to −0.18 (Table 2). For DSS, the standardized beta for black ethnicity was −0.50 with age, sex, and study site in the model and remained virtually unchanged when psychosocial and health-related blocks were added (−0.47 and −0.43, respectively), but when socioeconomic factors were added, the standardized beta decreased to −0.25 (Table 3). Literacy score (with imputed values) and education were the largest contributors to the reduction in standardized beta associated with black ethnicity. These results remained the same when raw literacy score was used and those with imputed literacy scores were excluded.
Table 2
Change in Black/White Standardized Beta for the Modified Mini-Mental State Examination (3MS)* with Sequential Adjustments for Demographic, Psychosocial, Health-Related, and Socioeconomic Characteristics in Health, Aging and Body Composition Participants
Standardized β
|
||||
---|---|---|---|---|
Characteristic | Model 1 | Model 2 | Model 3 | Model 4 |
Demographic | ||||
Black | − 0.43 | − 0.40 | − 0.38 | − 0.18 |
Age | − 0.10 | − 0.10 | − 0.10 | − 0.06 |
Female | 0.10 | 0.11 | 0.13 | 0.10 |
Pittsburgh | 0.08 | 0.07 | 0.06 | − 0.03 |
Psychosocial | ||||
Needs more emotional support | 0.003 | 0.006 | 0.006 | |
Poor/fair self rating of health | − 0.07 | − 0.06 | 0.007 | |
Lives alone | 0.02 | 0.02 | 0.017 | |
Religious faith important | − 0.03 | − 0.03 | − 0.11 | |
Center for Epidemiologic Study depression scale score (per point) (range 0–43) | − 0.10 | − 0.09 | − 0.05 | |
Health-related | ||||
Current smoker | − 0.06 | − 0.05 | ||
Drinks more than seven glasses per week | 0.04 | 0.007 | ||
Physically inactive | − 0.05 | − 0.02 | ||
Stroke | 0.02 | 0.015 | ||
Hypertension | − 0.01 | − 0.02 | ||
Diabetes mellitus | − 0.03 | − 0.03 | ||
Obese | 0.004 | 0.02 | ||
Socioeconomic | ||||
Education | 0.36 | |||
Literacy (Rapid Estimate of Adult Literacy in Medicine score (range 0–66) per point) | 0.18 | |||
Family income (per income category) | − 0.05 | |||
Financial inadequacy (per inadequacy) | − 0.01 |
Table 3
Change in Black/White Standardized Beta for the Digit Symbol Substitution Test* with Sequential Adjustments for Demographic, Psychosocial, Health-Related, and Socioeconomic Characteristics in Health, Aging and Body Composition Participants
Standardized β
|
||||
---|---|---|---|---|
Characteristic | Model 1 | Model 2 | Model 3 | Model 4 |
Demographic | ||||
Black | −0.50 | −0.47 | −0.43 | −0.25 |
Age | −0.17 | −0.17 | −0.17 | −0.15 |
Female | 0.15 | 0.16 | 0.17 | 0.17 |
Pittsburgh | 0.18 | 0.17 | 0.16 | 0.08 |
Psychosocial | ||||
Needs more emotional support | 0.01 | 0.01 | 0.01 | |
Poor/fair self rating of health | −0.10 | −0.09 | −0.04 | |
Lives alone | 0.02 | 0.03 | 0.03 | |
Religious faith important | −0.29 | −0.03 | −0.02 | |
Center for Epidemiologic Study depression scale score (per point) (range 0–43) | −0.09 | −0.09 | −0.06 | |
Health-related | ||||
Current smoker | −0.07 | −0.07 | ||
Drinks more than seven glasses per week | 0.04 | 0.01 | ||
Physically inactive | −0.05 | −0.02 | ||
Stroke | −0.03 | −0.04 | ||
Hypertension | −0.03 | −0.02 | ||
Diabetes mellitus | −0.03 | −0.02 | ||
Obese | −0.03 | −0.01 | ||
Socioeconomic | ||||
Education | 0.26 | |||
Literacy (Rapid Estimate of Adult Literacy in Medicine score (range 0–66) per point) | 0.16 | |||
Family income (per income category) | −0.08 | |||
Financial inadequacy (per inadequacy) | −0.01 |
Findings from the multivariate models with cognitive test score as the dependent variable, adjusted by covariate blocks, are presented in Figures 1 and and22 for 3MS and DSS, respectively. Each bar represents the least squared mean score after sequential adjustment. Adjustments for psychosocial and health-related characteristics explain little of the difference between scores, but adjustment for socioeconomic characteristics (along with demographic variables) greatly decreased score disparities between black and white participants. The 7-point difference in 3MS score diminished to 2 points. In the model adjusted for characteristics from all four blocks, the 7-point difference diminished to 1 point, meaning that these characteristics explained 86% of the score difference in the fully adjusted model. Similarly, a 14-point difference in DSS score was reduced to 5 points, with 64% of the difference explained by adjustment for characteristics from all four blocks.
Modified Mini-Mental State Examination score in the 3,062 black and white Health, Aging and Body Composition participants: the effect of multivariate adjustments. SES = socioeconomic status.
Digit Symbol Substitution Test score in the 3,032 black and white Health, Aging and Body Composition participants: the effect of multivariate adjustments. SES =socioeconomic status.
A score of 80 points or below was used to evaluate low 3MS score for Health ABC participants. The black participants were seven times as likely to have low 3MS scores as white participants (odds ratio (OR) = 7.64, 95% confidence interval (CI) = 5.66–10.30). Subsequent models included adjustment of demographics and the addition of one of the three blocks: socioeconomic, health-related, and psychosocial. After controlling for demographic factors, the odds of low 3MS score for black participants compared with white participants increased to 8.23 (95% CI = 6.01–11.27). The odds of low 3MS score for black participants compared with white participants decreased slightly when health related or psychosocial characteristics were added (OR = 7.59, 95% CI = 5.47–10.55 and OR = 7.20, 95% CI = 5.19–9.96, respectively). When socioeconomic indicators were added, the OR of low 3MS score for black participants reduced substantially, to 2.53 (95% CI = 1.77–3.64) compared with white participants.
DISCUSSION
Consistent with previous work,1–4 the current study found that older black adults scored worse on cognitive function tests than age-comparable older white adults but that, after accounting for differences in demographic and socioeconomic characteristics, score differentials decreased markedly. Characteristics not commonly measured in studies of cognition and dementia, such as literacy and financial adequacy, in addition to age, sex, and education, were significantly associated with cognitive test scores and helped explain differences in scores between older black and white adults. The score differential diminished to a greater extent for the 3MS than the DSS test, possibly indicating that socioeconomic indicators affect deficits in cognitive domains such as memory and language more than attention and visual spatial abilities do.
The magnitude of the ethnicity-related disparity in cognitive performance in this study was comparable with previous studies of older black and white adults that found lower cognitive test scores for blacks on the Mini-Mental State Examination (MMSE), Short Portable Mental Status Questionnaire, Community Screening Interview for Dementia, and the Buschke Verbal Memory Test.2,3,8,21–23 Several studies have attributed such differences to differences in level and quality of education.9,24–27 Indeed, one study that included black and white participants with similar education levels reported similar cognitive test scores.28 More recently, a study on reading level and cognition in black participants found that reading was a significant predictor of cognitive scores independent of education, suggesting that quality of education may be associated with differences in cognitive performance.8,9 The current study suggests that literacy, a correlate of education and possibly a more accurate measure of reading abilities over a lifetime, is strongly associated with cognitive test performance and mediates some of the differences between cognitive scores between older black and white adults. The adjustment of socioeconomic indicators greatly reduced the difference between black participants and white participants in odds of low 3MS score, defined using a cutoff of 80 on the 3MS.
This study builds on prior work including a wide range of psychosocial, health-related, and socioeconomic characteristics. Few studies,7,27,29 have simultaneously evaluated socioeconomic and health-related differences as potential mediators of cognitive test score differentials across minority groups. The current findings clearly indicate that socioeconomic factors such as education, literacy, income, and financial adequacy not only have an independent association with cognitive performance, but also explain a substantial amount of the difference in cognitive testing between older blacks and whites. Socioeconomic factors explain much more of the difference than any of the health-related factors examined. Given that the 3MS and DSS and similar batteries are routinely used to evaluate the cognitive status of older adults and may often form the basis for clinical treatment and placement decisions, attention to non-health related factors that may affect performance on these tests is even more critical. In both blacks and whites, education, income, and disease are potentially modifiable, and lower scores should improve in both groups as these disparities are addressed over time.
Prevalence30,31 and incidence3,32 studies of cognitive impairment typically find that blacks have higher rates than whites. Several studies of dementia or Alzheimer’s disease (AD) have attempted to discern whether the differences in disease rates are due to genetic or environmental causes. Most of these studies have used the MMSE to screen individuals and followed-up the screen with a clinical evaluation of dementia. Some studies suggest that the apolipoprotein E epsilon 4 (ApoE-ε4) allele is not as robust a risk factor in older black and Latino adults as it is in older white adults,33 but other studies report similar rates of dementia by ApoE-ε4 status in primarily white34 and primarily black groups. A cross-cultural study performed in Nigeria and Indiana found that the incidence of dementia and AD was nearly three times higher in blacks from Indiana as from Nigeria. The authors speculated that high rates of vascular risk factors in Indiana, as well as differences in the association between ApoE-ε4 and AD, may explain the differential incidence rates, not differences in SES or literacy.35 Perhaps the issues of social standing and perceived poverty relative to other groups are as important as actual SES measures,36 thus explaining why SES per se did not predict greater AD incidence in Nigeria than in the United States. Consequently, the current findings—namely that low literacy is associated with poor cognitive function—must be viewed in the context of relative affluence of the United States compared with other countries.
Potential hypotheses regarding the effect of education on dementia37 can be extended to help explain why, in this study, socioeconomic indicators such as education and literacy accounted for most of the ethnoracial variation in cognitive function test scores. The first hypothesis is the selective hypothesis: people who function poorly on cognitive function tests have diminished cognitive reserve from early childhood. The second hypothesis is an associational hypothesis: poor education correlates with other potential mechanisms such as poor nutrition, alcohol abuse, and inadequate health care; these other mechanisms account for why minority older adults score lower on cognitive function tests. The third hypothesis is the educational hypothesis: lifelong education builds a cognitive reserve that could compensate for brain deterioration. Because the current findings suggest that education and literacy are potent predictors of the race/ethnic disparity in cognitive function test scores after controlling for many known confounders including comorbidities and alcohol, the educational hypothesis is the most likely.
Some potential limitations of this study deserve comment. Although adjustments were made for many potential mediators of cognitive performance that differed between blacks and whites, a few key confounders were missing. For example, there exists some evidence that lifetime stress negatively and occupational attainment positively influence cognition, but these factors were not measured here.38 Second, because this study is cross-sectional in design, one cannot determine whether factors associated with cognitive performance would also be associated with cognitive decline. Future studies will examine the role of these factors in cognitive change over time within this cohort. Finally, this study addresses cognitive test score differences between older black and white adults; further study is needed to address the relationship between ethnicity and cognition in ethnic groups other than black and white. In addition, further investigation is needed in people of varied socioeconomic status.
This study suggests that black participants had lower unadjusted cognitive function scores than white participants, but after adjustment for socioeconomic factors and other variables, the difference in scores between blacks and whites decreased substantially. In addition, characteristics not commonly measured in cognitive studies, such as literacy and financial adequacy, were found to be important predictors of cognitive scores. Specifically, tests that can assess scholastic aptitude in early life may be important in separating the effects of literacy, premorbid intelligence, and education on cognitive function test scores in diverse older adult groups. Future studies with detailed examination of literacy, scholastic aptitude, financial adequacy, and other socioeconomic factors over the life course are needed to investigate the mechanisms by which these factors affect cognitive test differences. In addition, further studies are needed to examine whether these same socioeconomic factors may help explain differences in rates of cognitive impairment or AD in people with or without genetic risk factors.
You may like
-
Video: Inside Asamoah Gyan’s $3 Million Mansion: A Luxurious Haven with Stunning Views
-
Video: Nana Tonardo Sprays Cash on Nana Agradaa During Church Visit
-
Juliet Ibrahim Champions Gender Equality with HER STEAM Initiative
-
Video: Funny Face Flees After Learning Lady Shares Name with His Ex
-
Video: Lisa Quama Revives Ghana’s Independence Spirit with Stirring Nkrumah Reenactment
-
Tiwa Savage Eyes Mogul Status with Bold Plans for Music and Film Industry Expansion
-
The 28th February Crossroads Shooting: The Spark That Ignited Ghana’s Independence
-
Mahama Vows to Usher in Era of Fiscal Discipline and National Renewal
-
Elon Musk changes his name to Kekius Maximus on X